DataOps: More Than DevOps for Data Pipelines
“Let’s redefine it: DataOps is an engineering methodology and set of practices designed for rapid, reliable, and repeatable delivery of production-ready data and operations-ready analytics and data science models.” – Eckerson Group
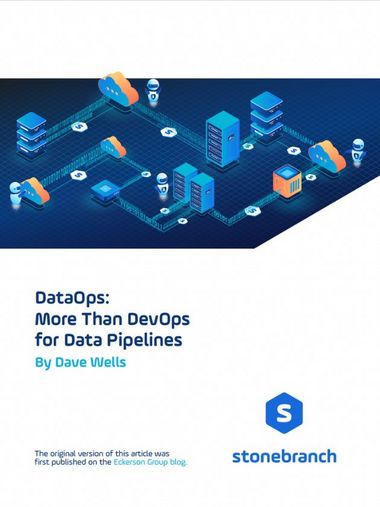
Data pipelines exist because today’s enterprises require analytics-ready data to make data-informed decisions. But how many DataOps definitions have you seen that even mention that true purpose?
This article by the Eckerson Group’s Dave Wells expands the definition of DataOps to integrate data pipeline and data science and analytics. But it doesn’t stop there — it puts both into action with a clear Continuous Integration / Continuous Development (CI/CD) methodology and a mandate for automation.
In this enhanced definition of DataOps, you’ll find:
- The true definition of DataOps vs DevOps, and how it’s so much more than just data engineering.
- The four dimensions you need to consider to succeed in your enterprise data operations.
- A diagram of the two interacting CI/CD loops that shape the DataOps methodology.
- A diagram of the key points of automation in DataOps dev/test/prod cycles.
- Key features to look for in data operations solutions that automate and orchestrate your entire big data pipeline.